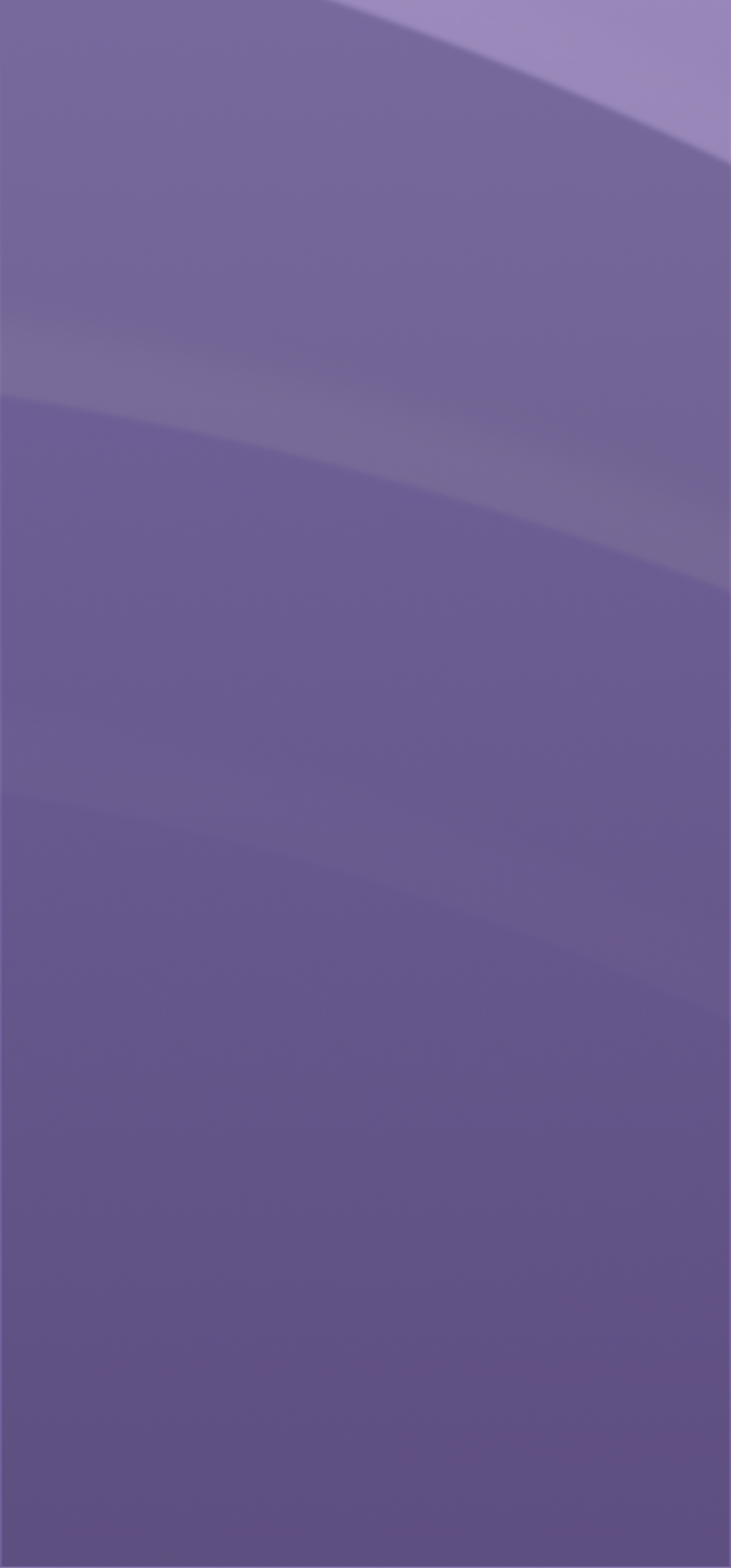
DeepSeek Disillusion: Evaluating the Future of AI
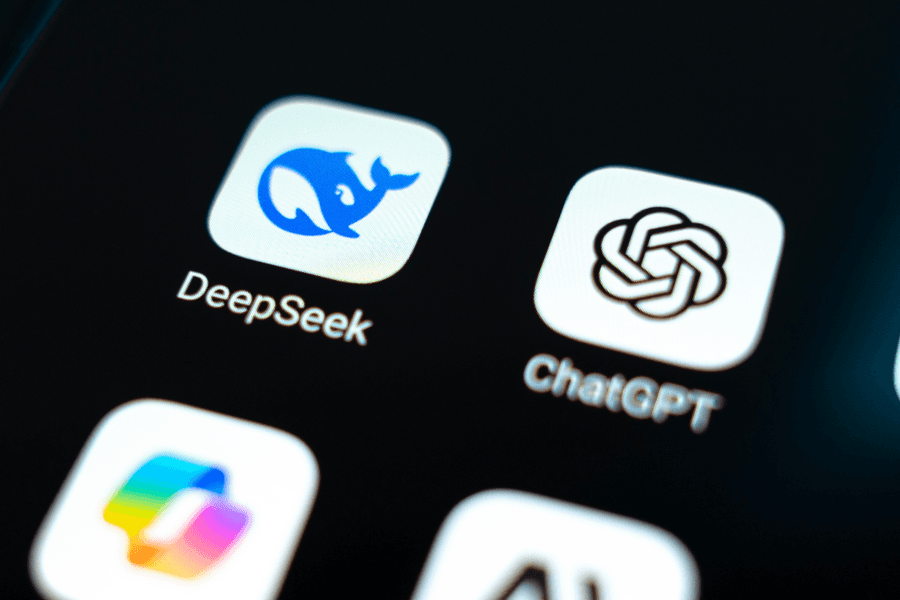
The release of DeepSeek has created a great deal of noise in the market. While many may see the news as a potential threat to the status quo of the artificial intelligence (AI) sector, innovations in AI such as DeepSeek, even if they cause temporary volatility, will most likely have a positive impact on investment opportunities in the space.
In this paper, we analyze the market reaction and evaluate the reality of how DeepSeek’s entrance may impact the future of investing in AI.
Background
In January, a claim by Chinese startup Hangzhou DeepSeek Artificial Intelligence Co., Ltd. that the training of its latest DeepSeek-V3 AI models required less than $6 million worth of computing power sparked a sharp sell-off in semiconductor stocks like NVIDIA Corporation and Broadcom Inc. The underlying assumption driving this sell-off was that DeepSeek’s efficiencies would dampen enthusiasm for AI infrastructure spending. Networking and power stocks slumped for similar reasons. Software stocks, on the other hand, rose on expectations that their margins and demand for their AI products will improve as AI becomes cheaper to run. Although NVIDIA and other stocks hit by the news quickly regained some of their losses, the impact of DeepSeek is still being weighed.
Was this news truly as earthshattering as the market seemed to believe at first glance? In our view, the market’s reaction to DeepSeek was overexaggerated. As SemiAnalysis, a well-known independent research and analysis company, noted, “To close watchers of the space, this is not exactly new news… When the world finally paid attention, it did so in an obsessive hype that doesn’t reflect reality.”
Yet we are also not surprised. We think the market was skittish for three reasons. First, last year, the market was characterized by the narrow leadership of the Magnificent 7. NVIDIA’s stock rose more than 170%. Private companies like OpenAI, Inc. and x.AI Corp. raised money at heady valuations. The latest OpenAI valuation was $157 billion according to public estimates, and the rumor is that OpenAI plans to raise another $40 billion at a valuation of $340 billion. X.AI was estimated at $50 billion in the latest round, double the valuation in the previous round. Many market observers considered the valuations of companies at the forefront of the AI revolution to be priced for perfection.
Second, the billions of dollars slated for the AI buildup continued to ramp. At the beginning of 2025, President Trump announced Stargate, a new AI infrastructure project in collaboration with OpenAI, SoftBank Group Corp., Oracle Corporation, and MGX Fund Management Limited, with a $100 billion initial investment that goes up to $500 billion over 4 years. Meta Platforms, Inc. raised its capital expenditure on AI plants to a range of $60 billion to $65 billion. The Street was expecting $50 billion. Satya Nadella, the CEO of Microsoft Corporation, said “I’m good for my $80 billion.”
Third, the media seized on DeepSeek as a major disruptor in the AI universe with hyperbolic, eye-catching headlines. Why did it blow up so much? Whether it’s CNBC, a podcaster, or a blog writer, the media makes money by getting the attention of its audience, and they get that attention by creating hysteria, debate, and questions.
The AI Arms Race
The North Star – the ultimate objective – in AI is artificial general intelligence (AGI), and artificial super intelligence (ASI). Achieving these goals will theoretically allow every industry in the world bottlenecked by intelligence – where the limiting factor is intelligence – to meet their objectives, or significantly expedite advancing toward their objectives. For example, in medicine, AI could help cure cancer. In neuroscience, it might help people with disabilities. On the extreme end, AI might help alleviate world hunger. If anything can be solved with AI, the thinking is it will happen. In the process, AI will unlock trillions of dollars in value for new markets and create value in existing markets.
"The North Star in AI is artificial general intelligence and artificial super intelligence."
The expectation, however, is not that AI will reach a certain point and everything will be resolved. Rather, it is a direction that AI is moving in.
At the forefront of the AI build out are the frontier model developers, such as OpenAI, Alphabet, Inc., Microsoft, and Anthropic PBC. Their objective is to come up with a breakthrough model with the best AI capabilities. These models will be evaluated against various benchmarks to determine which model performs the best – the frontier model. The expectation is that the model, once built out, will generate significant returns on the developer’s investment down the line.
The problem with this hoped-for outcome happens when the next model comes in six or nine months later and provides similar performance at a fraction of the cost. It could be another frontier model developer or a “fast follower” like DeepSeek, companies that quickly adopt and improve upon existing innovative AI technologies developed by others, rather than pioneering new ones themselves. The return on investment of the original frontier model builder is then eroded. That is the debate happening with DeepSeek now.
As for the frontier models themselves, there are two main types: proprietary models and open source models. Proprietary models, such as OpenAI, Google’s , x.AI’s Grok, and Anthropic, require a subscription for access. For example, OpenAI’s ChatGPT Plus is $20 a month and ChatGPT Pro is $200 per month. OpenAI also offers the option to charge via APIs, or pay per use for the actual data sent to and from the models.
With open source models, anyone can download and use them for free. A Meta Platforms, Inc. or a DeepSeek builds the model, and a model hosting company provides a platform to host and make it accessible for use in applications or services. Those with a large enough infrastructure can download the model onto their system and run them from there without the need for a host company.
DeepSeek in Context
The debate around DeepSeek centers on two claims. First, there is the assertion that DeepSeek conducted all the training of its model at a very low price -- just $6 million -- while the Western frontier model builders are deploying billions of dollars. This assertion raised the specter that fast followers like DeepSeek could compete with the frontier model builders at a fraction of the cost.
However, if you dig deeper, according to DeepSeek’s own statement, the $6 million figure referred just to the last run. There is much more involved in AI model training. It would be the equivalent of stating, “Out of 100 processes we employed to train the model, that one process cost $6 million.” If we believe DeepSeek’s statement, conservatively, it still would have spent hundreds of millions of dollars. If DeepSeek used more advanced chips than NVIDIA’s H800 chips as claimed, the cost could have been a billion dollars-plus.
The second claim is that DeepSeek is providing its service at an extremely cheap price. This is true -- the price is estimated to be 1/30th that of OpenAI. The cost of using DeepSeek via a model hosting platform is three to four times less than that of OpenAI. Of course, everyone wants cheaper AI. It is why software stocks went up on the news.
In the worst-case scenario for OpenAI, it is not making money while DeepSeek is, and OpenAI’s model is larger than DeepSeek’s for the same performance. However, that is just one possibility. It is important to ask what DeepSeek’s business strategy is in offering the service at such a low cost. Is it a loss leader in a bid to take market share? There are a number of other unknowns here that could play into this analysis.
It is also important to note that DeepSeek is not the only inexpensive large language model. Google’s models are even cheaper for the same performance. And while access to DeepSeek through its servers in China may be inexpensive, accessing the service in the U.S. costs significantly more.
Sputnik moment
Marc Andreessen, a well-known Silicon Valley venture capitalist, posted on social media that DeepSeek’s release was a Sputnik moment, referencing the Soviet Union’s 1957 launch of the Sputnik satellite into the Earth’s orbit, the first to do so. This event shocked Western nations, which had expected to be the first to achieve this feat.
Although the media quickly seized on this comparison, in the context of the historical pace of AI investments, DeepSeek was not nearly as significant a breakthrough as Sputnik. In fact, DeepSeek falls directly on the expected trend line for the cost of training an AI model.
However, from a geopolitical standpoint, the fact that DeepSeek came from China was impactful. Andreesen’s analogy appeared to imply that Western nations had assumed they were far ahead of the rest of the world on model development. With DeepSeek, they realized other parts of the world are closer than they thought.
Sputnik ushered in the space race between the U.S. and Soviet Union, with the U.S. accelerating its investment in space exploration. We expect a similar reaction from the frontier model companies. It is still early and nobody wants to lose this race. If the gap with other countries is smaller than they anticipated, we would expect the frontier model companies to ramp their investment in AI even more.
Standard vs. Reasoning Models
AI comes in many different guises. Two major types of AI models are standard and reasoning. A standard model focuses on predicting the next word in a sequence based on patterns in the data on which it has been trained. It is similar to knowing the answer in one’s gut. For instance, when people are asked “what is two times two,” they do not do the calculation; rather, they simply respond, “four.” Chat GPT-IV and DeepSeek-R1 are standard models.
Reasoning models go beyond simple text generation and aim to simulate logical reasoning processes, often including an intermediate “thinking” process. Shortly after DeepSeek was released, OpenAI released the latest iteration of its reasoning model, the o3-mini. The performance of OpenAI’s o3-mini is much, much better than its first reasoning model, o1. It also suggests we have already left DeepSeek-R1 behind.
At the same time, OpenAI launched a new product, Deep Research, which is an AI-powered agent designed to conduct in-depth, multi- step research on the internet. Unlike standard ChatGPT browsing capabilities, which provide quick responses, Deep Research autonomously finds, analyzes, and synthesizes information from hundreds of online sources.
An agent like Deep Research is comprised of three parts. First, the AI model, which is o3 in this case. Second, the tool used to interact with the world. This can be anything, like a calculator or a browser. Third is orchestration, where the agent uses reasoning to synthesize input and complete multi-step research tasks. As the name suggests, Deep Research retrieves all the information available online, synthesizes it, and creates a research paper in 10 or 20 minutes. While it cannot access paywalled content, including most scientific papers from subscription journals, it can work with any information readily available on the open internet and any files uploaded to it.
Semiconductors and Infrastructure
Semiconductor stocks like NVIDIA were initially hit hard by DeepSeek on the assumption that its efficiency improvements would dampen demand for chips and infrastructure buildout. This assumption is misplaced.
The improvements seemed new because, until now, there had been little attention paid to how AI was evolving since the advent of OpenAI in November 2022. It gave the sense that for the last two years, nothing changed. But a deeper dive into the academic literature confirms that what DeepSeek was able to do is not new. The DeepSeek improvements, whether on the architecture level or the software and hardware level were already well known among close observers of the space prior to the release of DeepSeek.
In addition, the drivers of data center demand remain intact. These drivers can be divided into two phases: training and inference. The training phase is the primary driver at this stage in the development of AI. The idea is that the more data a model is trained on, the more intelligence it will have. These scaling laws have held and it is believed they will hold in the near future, so the build out should continue regardless of DeepSeek.
In the long term, the inference phase will drive data center demand. Ultimately, it is believed that every workload, especially in the digital world, will be powered by an AI model at the backend. Again, it does not seem to us that DeepSeek will reduce that inference-based demand, given the innumerable use cases for AI. In fact, on the inference side, DeepSeek, with its promise of cheaper access to AI, ignited the imagination of edge use cases.
|
Use of AI in Software Applications
Virtually every enterprise software company today is looking into or actively integrating AI into their workflow, and AI is becoming increasingly customized for different functions. The most successful applications thus far have been large platforms that are well integrated within the enterprise. Microsoft Corporation is a good example. The company has estimated that it will generate $13 billion in revenue from AI derivatives. ServiceNow, Inc., one of the most successful enterprise software companies, has reported that its AI product Pro Plus is selling faster than any other product in its history.
Agentic AI
Pro Plus is an example of agentic AI, or an AI agent. Where Generative AI (Gen AI) can create original content—such as text, images, video, audio or software code—in response to a user’s prompt or request, agentic AI describes systems that are designed to autonomously make decisions and act, with the ability to pursue complex goals with limited supervision. It is a proactive AI-powered approach, whereas Gen AI is reactive to the user’s input. Agentic AI can adapt to different or changing situations and has “agency” to make decisions based on context. It is designed for applications that can benefit from independent operation, such as robotics, complex analysis, and virtual assistants.
For example, for a sales representative looking to cold call prospective clients, a Gen AI product could potentially write an email. An AI agent, on the other hand, can potentially identify all the prospects in the funnel and send the email itself. It will try to do more than just answer questions but take action, which is the next level of productivity. This next step will happen more slowly in enterprise because the cost of a mistake is relatively higher.
Today most AI models are aimed at the average consumer. In the future, we will likely have more sophisticated models for specific industry or business purposes, with the aim of selling the solution and not the model itself. The solution may be as simple as a note taking app or as advanced as a cure for a disease.
We are already seeing that start to happen. There are rumors that Anthropic has a very large model, but it is not releasing it. It would rather have a smaller fine-tuned or distilled model at a lower cost than release its frontier model to the world.
Small language models
Unlike AGI or ASI models that can do everything, companies are developing smaller domain or industry-specific models that are trained on and specialized for domain-specific or customer data. As the model gets larger and more sophisticated, it costs more. However, in specific use cases, much of that data may be irrelevant. For example, an application that takes notes in meetings does not need to remember the history of Japan to summarize those notes. To save money and create efficiencies, enterprises are building small language models or SLMs that know to identify what is important in a sentence or in a paragraph. It tries to do only what is needed to improve the unit of economics.
Today most AI models are aimed at the average consumer. In the future, we will likely have more sophisticated models for specific industry or business purposes, with the aim of selling the solution and not the model itself. The solution may be as simple as a note taking app or as advanced as a cure for a disease.
We are already seeing that start to happen. There are rumors that Anthropic has a very large model, but it is not releasing it. It would rather have a smaller fine-tuned or distilled model at a lower cost than release its frontier model to the world.
Physical AI
Yet another type of AI is physical AI, the fusion of AI with real world sensor data, enabling real time perception, understanding, and reasoning about the physical world. Examples are self driving cars and robots. It differs from large language models in two major ways: input and safety. While a user interacts with a large language model largely through text or voice, physical AI consumes data mostly from sensors. It needs to understand what is around, determine what is important, and make decisions. It’s very different than summarizing a paragraph or providing a recommendation for a vacation in Italy.
The second difference is the safety issue. A wrong recommendation for a trip to Italy is far less risky than a car making a wrong turn or a surgical robot cutting the wrong artery. This safety factor means the level of accuracy must be significantly higher.
As an example, Tesla, Inc. is employing physical AI to develop robotaxis and humanoids. Its Dojo supercomputer is designed for AI training of its machine learning models for its Full Self-Driving system. Dojo’s goal is to efficiently process millions of terabytes of video data captured from real-life driving situations from Tesla’s 4- plus million cars.
Full Self-Driving will enable Tesla to produce RoboTaxi fleets that will deliver significantly more value by increasing the utilization of individual cars. The cost of a car is $35,000 whether Tesla sells it or employs it as a RoboTaxi. A single owner car is driven about 10 hours a week. There’s at least 50 to 55 more hours of utilization you could get from that car as a RoboTaxi. This market could generate much more profit and revenue than the traditional automotive market.
Around 11 trillion miles are driven every year globally; 3.3 trillion in the U.S. At a dollar a mile, this could translate to significant revenue.
With its Optimus humanoid robot, another manifestation of physical AI, Tesla is leveraging the same batteries, compute, and cameras developed for its vehicles. Humanoids have an advantage over autonomous vehicles in that a humanoid can gather significantly more data than a car because it can do more tasks. Tesla’s goal is to build humanoids at scale. Tesla expects to produce thousands of humanoids this year, potentially 5 to 10 times as many next year, and scaling from there.
Has NVIDIA Peaked?
NVIDIA has likely not yet peaked, in our view, although expectations seem to have caught up with it. The inflection in NVIDIA’s business was astonishing, comparable perhaps only to Apple Inc. in the early days of the iPhone. We believe NVIDIA will remain a leader, but it is unlikely that it will see returns similar to those of the past two years.
Looking ahead, the challenge for NVIDIA is how large the market will be. NVIDIA currently holds more than 90% of the market for AI systems. A significant percentage of this market in the race for AGI and ASI will likely continue to go to NVIDIA, but it is also competing with custom players such as Broadcom.
Broadcom has three major customers in the accelerator space. One is Google and the other two are rumored to be Meta and ByteDance. Broadcom’s play with the hyperscalers is that, for customers who know what their end product or app, model, and underlying software will look like, Broadcom can help optimize across all the layers – building the hardware, software, and application systems.
Broadcom is a leader in Tensor Processing Unit, or TPUs, which are similar to NVIDIA’s GPUs but customized. TPUs optimize performance and cost for AI workloads, from training to inference. All the training for Google’s models uses TPUs. These models are offered at a huge discount. While there is some business strategy involved, it is mostly because Google owns the entire stack. Other players with similar plans also want that control, not just to save money but to ensure independence. When a company is spending billions of dollars building a model, it needs to have its own path carved out. It cannot be beholden to someone who is the gatekeeper of the hardware.
With Broadcom having showcased that it can successfully partner with companies like Google and Meta, it has built the credibility and instilled the confidence it can do the same with other companies. Over the next few years, while AGI continues to build out, we see Broadcom as the best positioned to leverage that opportunity.
How Will DeepSeek Impact Meta and Google?
While DeepSeek will likely not impact either business, continuing advancements in AI will likely impact both. Google’s models are leading frontier models and Meta’s Llama is a leading open source model. With its core product, Search, Google has 90% market share of one of the greatest businesses ever invented. That business will change over time. In the future, users will want more than just the familiar 5 or 10 blue links. They will want answers and agents like Deep Research to perform tasks for them. This will be monetized in a different way, so while Google Search is under threat, it is not because of DeepSeek.
In addition, we see more potential competition from both the high end and the low end. On the enterprise software level, every time there is a tectonic shift in technology, there is opportunity for new players. For example, the echelon companies founded in the cloud era effectively took most of the market from the legacy software providers. This could happen with AI, where AI natives will have leaner cost architecture, have better talent, and be able to innovate faster.
The software providers best positioned capitalize on AI, versus those that will be disrupted, already have the advantages of a robust distribution network and scale and differentiated data. Companies with a sizable existing customer base will be able to sell add-ons fairly easily. Scale is also important, because it gives companies the capacity to invest in R&D and purchase pricey GPUs. Lastly, differentiated data, either their own or that of their customers, can be used to train a model to provide enhanced solutions to their customers. But again, there are no guarantees. As all the legacy competitors are trying to do the same thing, it is important to monitor competitive dynamics.
Conclusion
DeepSeek was an important breakthrough in AI research and development. It demonstrated that not all high-performance AI has to come with an exorbitant price tag, challenging conventional economic models and fostering innovation across multiple industries.
At the same time, DeepSeek’s improvements were already well known to close observers of the space prior to its release. It is just one of numerous innovations happening across the AI landscape as the most important development in technology since the advent of the internet continues to evolve at a rapid pace.
Featured Products
Baron Opportunity Fund
- InstitutionalBIOIX
- NAV$43.38As of 04/23/2025
- Daily change2.87%As of 04/23/2025
Baron Technology Fund
- InstitutionalBTECX
- NAV$11.33As of 04/23/2025
- Daily change3.47%As of 04/23/2025