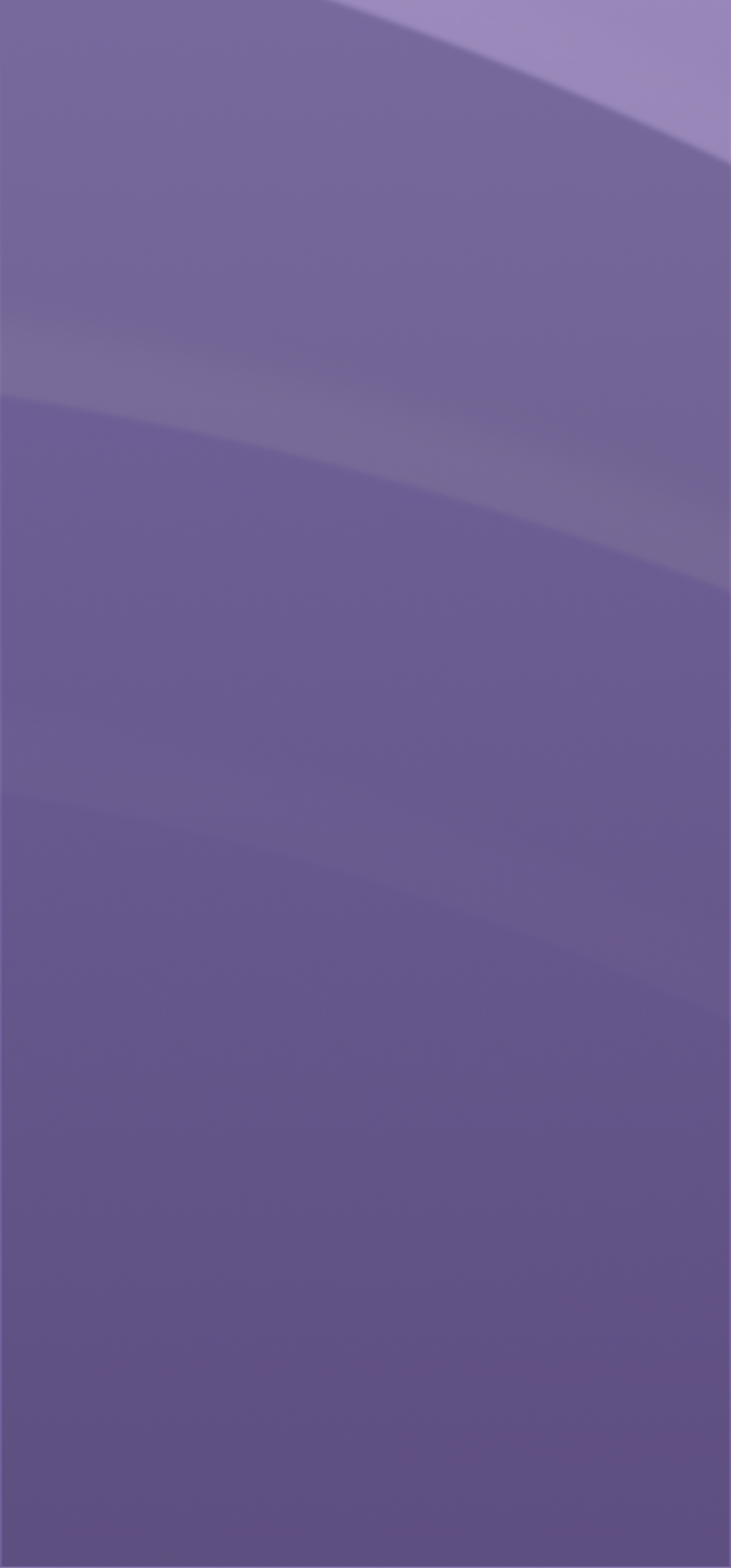
AI Hype and the Death of Software
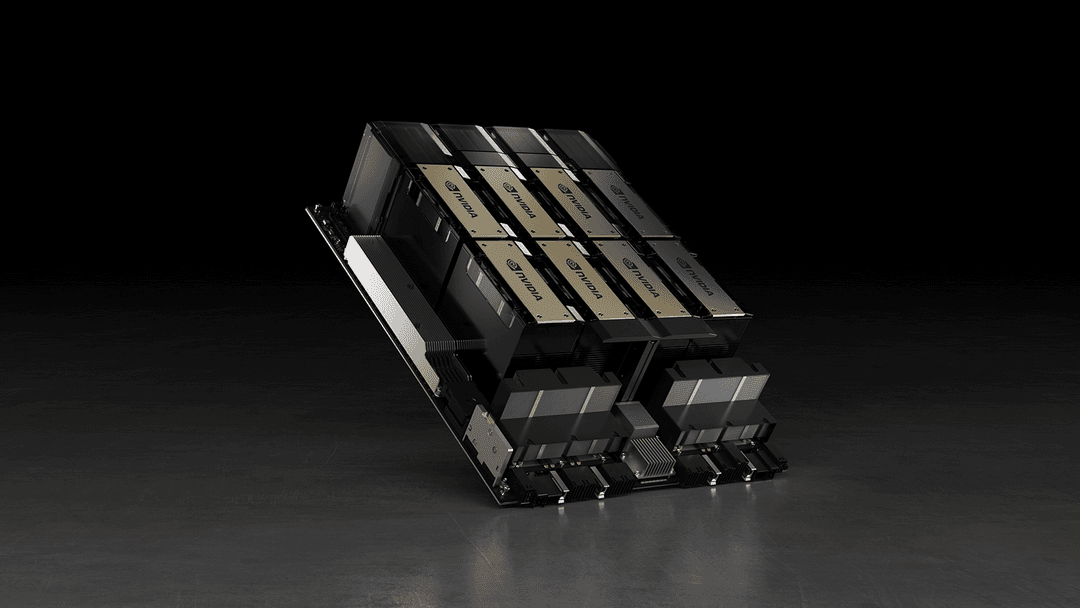
NVIDIA Corporation
AI Hype vs. Reality
Less than two years ago, in late November 2022, the public introduction of ChatGPT unleashed a generative AI (Gen AI) frenzy. Stocks of expected beneficiaries, led by the newly coined Magnificent 7, soared to eye-popping valuations, dominating the global markets to a degree unprecedented in history.
Fast forward to the present and observers are questioning whether the excitement around AI is justified or simply irrational exuberance and destined to end badly for the investors who climbed aboard the AI bandwagon.
The reality is somewhere in between.
We do not dispute that there is some hype around AI and the perceived AI winners. History teaches that there is always a hype cycle around major technology disruptions–initial euphoria, a short period of doubts and questions, and then the measured reality of the true impact the shift is having. Yet, hype aside, we believe AI is the most significant advancement and technological platform shift impacting our now-digital world since the advent of the internet itself in the mid-1990s, some 30 years ago.
The AI hype cycle
Like many new technologies, Gen AI has been following a path known as the Gartner hype cycle, first described by U.S. tech research firm Gartner.
This widely used model describes a process that frequently follows the introduction of a new technology. After the early “peak of inflated expectations” comes a “trough of disillusionment,” followed by a “slope of enlightenment” which eventually reaches a “plateau of productivity.”
We have been experiencing almost a classic hype cycle following the ChatGPT moment in late November 2022. Most of this time has been a period of euphoria prompted by the initial introduction and adoption of AI consumer and business applications, announcements and public data releases regarding the improvements in large language models (LLMs), as well as the historic inflection in sales of GPUs1, otherwise known as accelerated computing chips, as reflected in the financial results of companies like NVIDIA and Broadcom. More recently, we’ve entered the period of doubts and questioning, some of which is real and normal in the first stages of a new paradigm, and some of which is prompted by short sellers. Given the explosive returns of NVIDIA and other AI leaders, AI bears and fear mongers have been comparing the current AI market winners with the internet bubble of the late 1990s/early 2000s, and NVIDIA’s stock move today with Cisco’s back then. We think this comparison is flawed.
First, while many stocks were trading at nosebleed valuations and on made up metrics (such as price per eyeballs) before the bursting of the internet bubble, the internet ultimately proved to transform our world and create the digital age we are now living in.
Second, we are not in a valuation bubble akin to the internet boom. As an example, while NVIDIA’s stock price inflection has been nothing short of unprecedented for a company of its size, it was fueled almost entirely by explosive growth in revenues, earnings, and cash flows – not multiple expansion. Over the last 12 months, NVIDIA’s stock has effectively tripled, but its forward P/E multiple has remained essentially flat, because NVIDIA blew away Wall Street expectations despite being covered by over 60 sell-side analysts, who have increased their forward projections every single quarter. In the last two decades, the only comparative analogue is when Apple first introduced the iPhone in 2007 and stunned Wall Street with its growth.
In contrast, most of Cisco’s move in the late 1990s was due to multiple expansion. At its peak, Cisco traded at a P/E ratio of over 130 times, more than quadruple its five-year average of 37 times. At the end of the second quarter, NVIDIA traded at a P/E ratio of 40 times, equal to its five-year average, and at a P/E to growth (or PEG) ratio for 2025 of 0.8 times, as consensus expectations are for NVIDIA to grow earnings per share 40% next year.2
Investor concerns have also arisen about the financial impact AI is having and whether surging capital expenditures (capex) across the technology landscape, particularly the large cloud players (Microsoft, Google, Amazon, and Meta), known as the hyperscalers, will be justified and earn reasonable returns on invested capital (ROIC). Again, we think these concerns are misplaced.
Technology Leaders on Gen AI
“[Gen AI is] the most important advance in technology since the graphical user interface [in 1980].” - Bill Gates, founder of Microsoft
“Generative AI is the most powerful tool for creativity that has ever been created. It has the potential to unleash a new era of human innovation.” - Elon Musk, founder of Tesla and SpaceX
“Generative AI is the key to solving some of the world’s biggest problems, such as climate change, poverty, and disease. It has the potential to make the world a better place for everyone.” - Mark Zuckerberg, founder of Meta (Facebook)
First, the adoption and penetration of new technology typically traces a classic S-curve–or more precisely, a series of S-curves or phases. For at least the past year and a half, we’ve been in what might be called the AI infrastructure build phase – building the AI factories, as NVIDIA CEO Jensen Huang has articulated it,3 and this phase has been dominated by the infrastructure-layer players – the accelerated computing chips suppliers like NVIDIA and Broadcom, as well as data center, cloud infrastructure and energy companies. The hyperscalers, other enterprises, and sovereign entities investing ahead understand that if you want to be in the AI game, you must invest now – build the infrastructure, build the factories – or else you’ll find yourselves disrupted on the sidelines or playing catch up in the biggest game, the most important race in a technology generation. Only those who invest today even have the chance to be the winners of the future.
While AI technology is new, the investment paradigm is not – upfront investments to generate long-term returns. All AI services of the future will require an AI factory, whether you own or rent one. The four hyperscalers mentioned above, among others, are leading the charge to build these AI factories. The four are expected to spend almost $200 billion on capex this year, a 32% increase over the amount the group spent last year. If you exclude Amazon, which doesn’t break out its data center capex from its fulfillment capex, the growth rate is 40%. Valuing and calculating the expected returns of a growth opportunity, like AI, which requires heavy investment, can only be done by examining and projecting the long-term opportunity.
To that point, we are in the earliest, almost preliminary stages, of what might be called the AI application phase. This phase – like the early days of the desktop or mobile internet eras – will take years. As in every prior technology generational shift, some early applications will have an immediate measurable impact, while many applications will fail, and others will require iterations and not be ready for prime time until the 2.0 or 3.0 release. Most companies are still in the proof-of-concept stage while very few are ready for production today. AI is developing rapidly across industries. Near term, there is a lot of excitement around AI for areas such as consumer chatbots, AI-based customer service, AI-based assistants for a variety of business tasks such as coding, marketing, back office, and more.
AI in ActionIn its report, The State of AI in 2023, McKinsey found 33% of businesses already use AI tools to cut costs. Another 12% reported using this technology to create new income streams. For example: | |
---|---|
![]() | Global financial institution ING developed a Gen AI chatbot that was able to assist 20% more customers than its existing chatbot. The new chatbot, first launched in the Netherlands, is expected to reach 37 million customers a year as ING rolls it out across ten markets. |
![]() | Buy now, pay later platform Klarna cut its payroll by more than 1,000 jobs and expects to shed 2,000 more, totaling 60% of its workforce, through its use of AI in customer service and marketing. Average resolution time shrank from 11 to 2 minutes while customer satisfaction scores remained unchanged. |
![]() | WPP, the world’s largest ad agency, deploys Gen AI to create ads, cutting marketing costs and expanding their reach. For example, the agency used AI to virtually recreate an African landscape at a fraction of the cost of sending a film crew to the location. |
A handful of reported AI applications and use cases have already yielded measurable impacts and ROIC. For example, in software development, AI services (from companies like Microsoft, Amazon, and GitLab) – such as code writing, revisions, documentation, vulnerability inspection, etc. – have provided meaningful productivity enhancements, with reports of 30% to 60% improvements in developer efficiency.4
In customer service, Gen AI chatbots can handle up to 80% of routine customer inquiries, freeing up human agents to focus on more complex issues and saving companies 15% to 30% on customer service operating costs.5 For the consumer internet players (like Meta, Google, TikTok), AI investments have improved core content and advertising platforms – algorithm and bid-rank improvements, more accurate targeting models, increased video engagement, dynamic ad insertion, and more – and have generated impressive ROIC.
Looking forward, published general economic studies have shown that up to $2.4 trillion in capitalized AI investments could generate a 25% ROIC with either operating expense reductions amounting to 5% of global skilled payroll or 3% of global total payroll or revenue generation at levels of 3% of global public company revenues or 2% of global GDP.
On the operating expense side, for example, eliminating one software developer would provide up to $250,000 of value or cost savings; cutting one knowledge worker from a team might accrue up to $150,000.
From the revenue generation angle, application-specific sell-side reports have demonstrated that, even at today’s pricing levels for AI services such as Microsoft Copilot or Azure AI Cloud, the returns on capital deployed and operating assets are material, though perhaps not as high as current generation cloud software or infrastructure services.6 On NVIDIA’s May earnings call, CFO Colette Kress boldly claimed that for every $1 spent on NVIDIA systems, a hyperscaler could “generate $7 in revenue over four years.”
We believe we are in the earliest stages of a multi-decade disruption. Longer-term avenues of development are broad and include drug discovery, in which the opportunity for AI is significant due to the long timelines for drugs to reach approval and the high probability of failure (90% of drugs fail); planning and running factories and supply chains using digital twins and AI simulation; and using AI to build robots across a variety of use cases (from autonomous machines to autonomous driving to humanoid robots). Multi-domain, multi-industry disruption.
When one considers where we are in AI today, and where we might be in a few years, one cannot ignore the pace of improvements we have already witnessed. Here are just a few examples of how quickly AI models are improving:
Accelerated computing chips
At NVIDIA’s June COMPUTEX conference appearance, Jensen Huang presented slides showing that AI compute had improved 1,000 times over the last eight years and energy use had improved 350 times.7 NVIDIA’s recently introduced Blackwell family of chips can produce performance improvements of up to four times faster for training and 30 times for inferencing compared to the prior Hopper generation. Blackwell can deliver 25 times lower total cost of ownership and energy consumption than Hopper, as well. The new Blackwell architecture provides the ability to combine a significant number of GPUs into a “single” large GPU (namely thanks to NVIDIA’s networking capabilities), and the company’s investor relations commentary stated that it expects Blackwell to be “the new unit of compute.”
Large language models
AI LLM’s algorithms are rapidly improving as well. For example, the price of OpenAI’s GPT-3, which cost $20 per 1 million tokens in early 2023, declined 95% with the introduction of the more capable GPT-3.5 Turbo, which costs $1 per 1 million tokens. In a recent AI publication8, the author presented his views and evidence that the “pace of [LLM] deep learning progress in the last decade has simply been extraordinary.” He argued that OpenAI’s “GPT-4 was merely the continuation of 10 years of breakneck progress in deep learning. A decade earlier, models could barely identify simple images of cats and dogs; four years earlier, GPT-2 could barely string together semi-plausible sentences. Now we are rapidly saturating all the benchmarks we can come up with. And yet this dramatic progress has merely been the result of consistent trends in scaling up deep learning…Another jump like that very well could take us to [artificial general intelligence], to models as smart as PhDs or experts that can work beside us as coworkers.” For GPT-4, released in 2023, he described it as a “smart high schooler” and commented: “Wow, it can write pretty sophisticated code and iteratively debug, it can write intelligently and sophisticatedly about complicated subjects, it can reason through difficult high-school competition math, it’s beating the vast majority of high schoolers on whatever tests we can give it, etc.” He presented this chart comparing GPT-3.5 and GPT-4 (models already two-to-three years old) to human test-takers:
GPT-4 (2023) | GPT-3.5 (2022) | |
---|---|---|
Uniform Bar Exam | 90th | 10th |
LSAT | 88th | 40th |
SAT | 97th | 87th |
GRE (Verbal) | 99th | 63rd |
GRE (Quantitative) | 80th | 25th |
US Biology Olympiad | 99th | 32nd |
AP Calculus BC | 51st | 3rd |
AP Chemistry | 80th | 34th |
AP Macroeconomics | 92nd | 40th |
AP Statistics | 92nd | 51st |
Source: Situational Awareness — The Decade Ahead, June 6, 2024, Leopold Aschenbrenner
Over time, as models continue to improve, and the cost of running them declines, an increasing number of human tasks could be augmented or replaced entirely by AI. Before long, every digital interaction–whether with business software, consumer apps, robots, cars, etc.–will be AI powered. AI will make humans more productive doing their jobs, developing drugs, designing products, writing software, being creative, and more.
Is Software Dead?
While AI demand and experimentation have clearly benefited semiconductors stocks (as well as certain energy, industrial, and data center stocks), it has created market uncertainty around the state of software. As depicted in the chart below, we have seen the widest discrepancy in semiconductor and software performance in 20 years.
Why has software lagged?
First, as discussed above, the AI application phase – where enterprise software lies – simply comes later on the AI S-curve or, as we see it, series of S curves. In our myopic, short-term focused market, there is less investor excitement for software, with most investors waiting to see the results – in contrast to the results they are seeing for, say, semiconductors – before jumping back into software. At the same time, some software applications (for instance, AI code writing tools) are already having a profound impact, while others are still in the proof-of-concept stage and will require further iteration and 2.0 or subsequent releases before they really impress.
Second, several software industry bellwethers, including Salesforce. com and Workday, reported soft financial results and issued disappointing guidance in their first quarter results released in May. Among the software companies we track, more than half guided their next quarter revenue below Street expectations. These companies cited a series of reasons including longer sales cycles and tighter IT spend environments.
While part of the weakness could be chalked up to IT budget cyclicality, the sudden shift does beg the question: is AI investment “crowding out” software spend? What does that mean for the long-term growth of software businesses? With CIOs and CEOs facing pressure to adopt AI technology and articulate AI strategies, a bearish narrative has emerged that some software models are at risk of being displaced or becoming obsolete.
As bottom-up investors, our investment goal for software, and any other industry vertical, is to own the winners, not the group. As with any technology platform shift – whether on-premise servers to cloud computing, desktop to mobile applications, or automation to intelligence – this means analyzing the threat of substitution, the change to competitive dynamics, and the impact on pricing models and unit economics.
While AI poses a risk to some software companies, we believe that, to paraphrase Mark Twain, the reports of its death are greatly exaggerated. The more likely explanation for the longer software sale cycles is that customers are being more thoughtful and strategic in their software vendor selection – they want to find the right longer-term partners whose products support their 3-5-10-year AI initiatives. Thus, we think the software businesses with the right architecture, product roadmaps, and customer value creation track records should see their competitive moats widen, not contract, as AI proliferates, and ultimately capture more IT budget share over time.
The enterprise software winners will have to be better at delivering AI services and features than build-your-own AI tools, and they will have to use their incumbency or leadership advantages to ward off upstarts. We believe the winners will have a well-established product development culture of innovation and iteration; differentiated proprietary, industry, and customer data; distribution advantages with large customer bases, successful go-to-market efforts, and key partners; well-designed workflows where AI improves the user interface, intelligent predictions/recommendations, and automation; and established always-on connectivity and feedback from their customers; among other things.
AI Winners in Software | |
---|---|
> Microsoft Corporation | Azure OpenAI – Microsoft’s suite of AI services that allows customers to apply natural language algorithms on data – is now used by 65% of the Fortune 100, and GitHub Copilot – Microsoft’s AI code writing service – is delivering 40%-plus improvements in developer productivity and already has 1.8 million subscribers. |
> Datadog, Inc. | A cloud observability platform used by the leading LLM providers to monitor their AI apps; these AI customers are already driving nearly $100 million of annual recurring revenue for Datadog. |
> Samsara, Inc. | This software platform for commercial vehicle fleet and physical operations management is applying AI to the proprietary data it collects from sensors embedded in over a million vehicles spanning billions of miles driven to prevent hundreds of thousands of accidents a year. |
Conclusion
As Bill Gates observed in his 1995 book The Road Ahead, “We always overestimate the change that will occur in the next two years and underestimate the change that will occur in the next 10. Don’t let yourself be lulled into inaction.”
We agree. Right on schedule, the AI hype cycle is shifting from the initial wave of enthusiasm, with its hyperbolic claims of all manner of changes, to disillusionment, similar to prior cycles such as the internet, mobile, and cloud. We see massive opportunities ahead over the next 10 years. The adoption and rapid improvement of AI capabilities will spur growth across many industries – not just the Magnificent 7 and other more obvious AI winners.
A related question is whether software is dead – an unattractive investment as companies shift IT spend to AI at the expense of software. We would argue that this view is too focused on the short term and to write off an entire sector is to miss out on select opportunities. We are still in the AI infrastructure phase, and the application software phase simply comes later in the S curve. Although AI poses a risk to some software models, we think software companies that successfully incorporate AI into their own solutions and leverage their leadership position or incumbency to fend off newcomers will flourish over the long term.
Contributors:
Ashim Mehra
Portfolio Manager, Baron Technology Fund/Strategy
Ishay Levin
Assistant Portfolio Manager, Baron Opportunity Fund, Baron High Growth Strategy
Brian Neigut
V.P., research analyst
Andrew Iftode
Research analyst
Featured Funds
Baron Opportunity Fund
- InstitutionalBIOIX
- NAV$44.87As of 04/01/2025
- Daily change0.99%As of 04/01/2025
Baron Technology Fund
- InstitutionalBTECX
- NAV$11.62As of 04/01/2025
- Daily change1.40%As of 04/01/2025