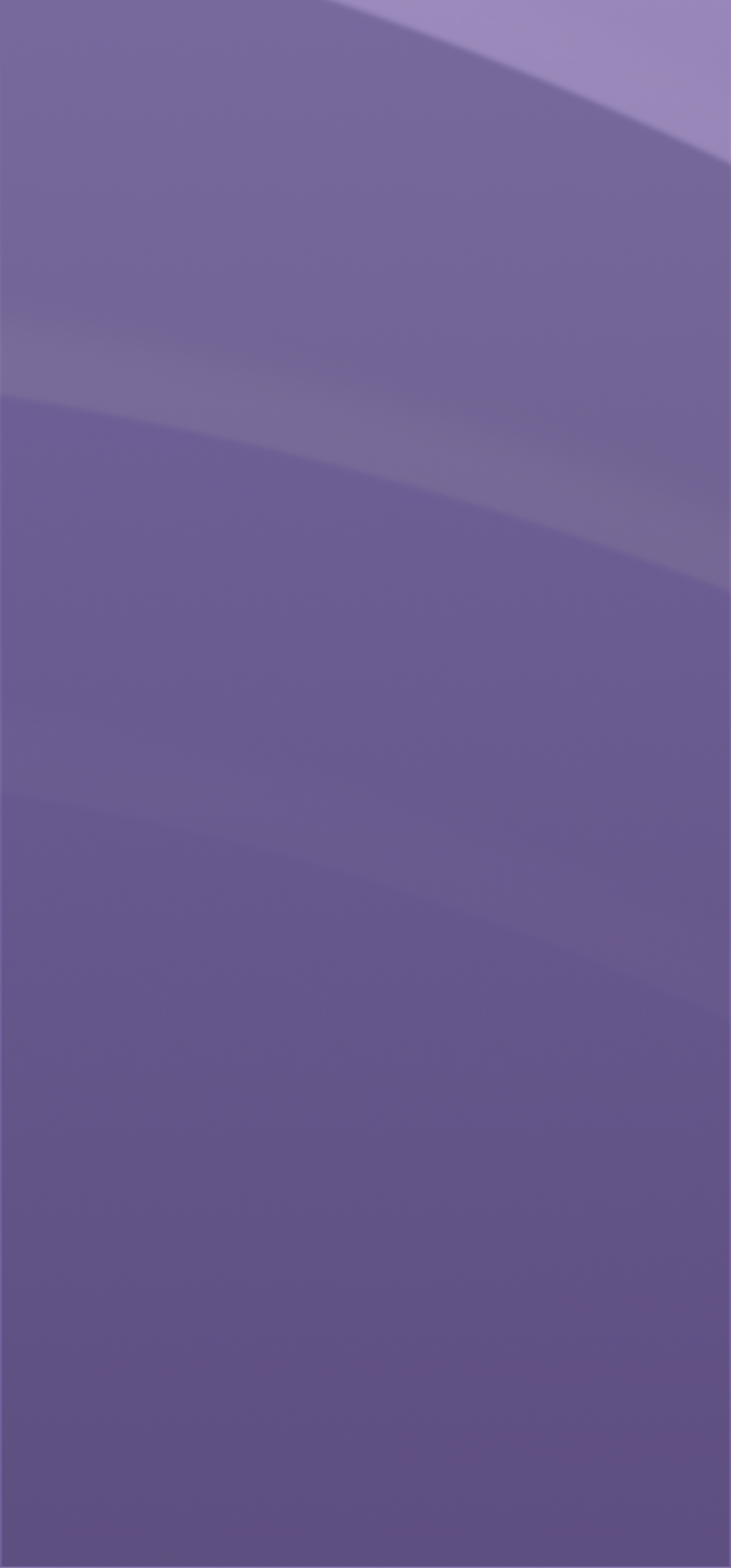
Agent Ascendancy: GenAI’s Future Frontliners in Large-Scale Consumer Applications
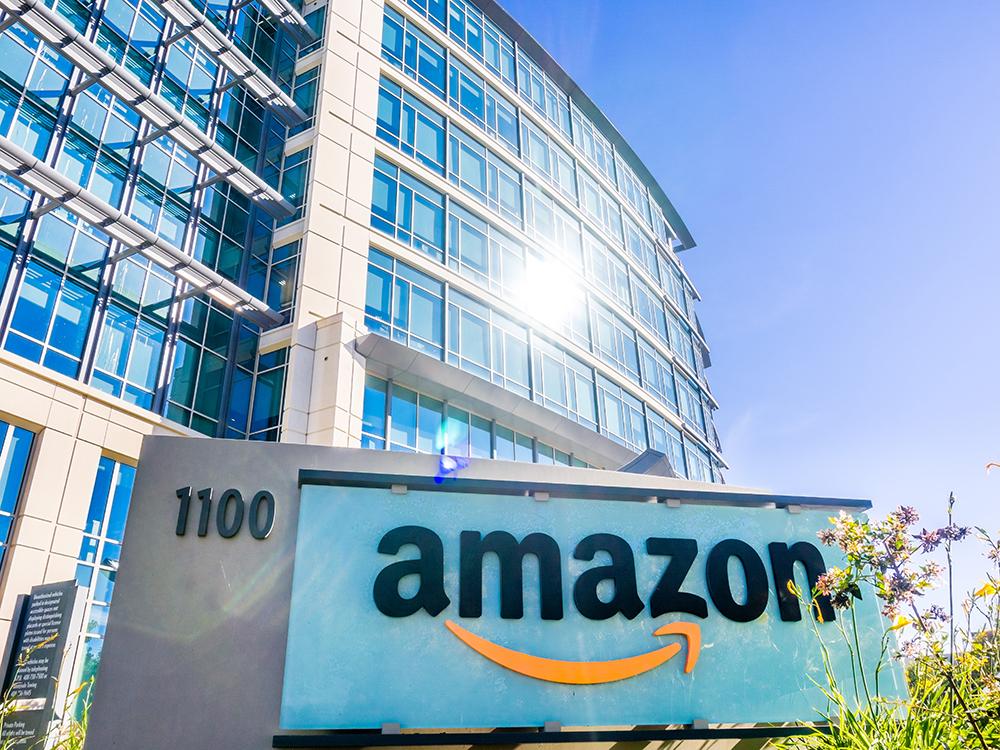
Amazon, Inc.
Overview
In this paper, we discuss how generative artificial intelligence (GenAI) will impact large scale consumer applications and which companies we believe are best positioned to leverage GenAI to their advantage over the next half decade.
We think GenAI will be the catalyst for the widespread use of AI agents (i.e., personal assistants) that will interact with users in a way that feels like a conversation with a superpowered live personal assistant rather than a machine. This marks a significant shift from how consumers currently interact with machines. We expect companies that embrace this new style of interaction model to benefit in this age of agent ascendancy.
Among all the large consumer application companies, we think four – Alphabet Inc., Meta Platforms, Inc., Amazon.com, Inc., and Tencent Holdings Limited — possess the long-term competitive advantages today that position them to transition into the leading GenAI consumer applications tomorrow. (While we have chosen to exclude it from this analysis, Microsoft Corporation owns 49% of OpenAI, the owner of Chat GPT, one of the largest GenAI consumer applications today. We expect Microsoft to be another major beneficiary of GenAI technology.) While there are other consumer application companies who will also successfully navigate this shift, we have confidence these four are exceptionally well equipped to excel.
To understand why, we first need to establish the foundation of the technologies on which these applications are being built. This foundation will provide the context for a better grasp of these companies’ competitive advantages based on their proprietary datasets, massive scale, and infrastructure.
We will begin by defining GenAI. We will then discuss where the AI agents/consumer layer fit in the broader technology stack. After that, we will explore how consumer use cases could evolve in the next decade. Finally, we will lay out the case for each of the four companies we have identified for success in this endeavor.
Introduction to GenAI
GenAI gained widespread consumer awareness following the launch of ChatGPT in November 2022. As a concept, AI is not new and, in fact, is used in everyday life across a wide array of applications such as virtual assistants like Siri and Alexa, personalized content recommendations on streaming platforms, and facial recognition software on cellphones.
What is distinctive about GenAI is the tremendous potential it holds to transform work across industries, creating efficiencies and boosting overall productivity. Taking a more holistic view, GenAI might not only bring the power of AI itself to the masses but also accelerate the democratization of innovation. Consumers could gain the ability to both create original content and manage everything from everyday tasks to complex projects by leveraging GenAI’s superior expertise and knowledge base.
To illustrate what this means, imagine if you taught a computer to recognize countless songs, stories, or images. After learning from all this data (today’s GenAI systems can scale and process over a trillion data parameters) the computer can produce an original song or image — something that has not been seen or heard before. As the perfect assistant, GenAI can also help enhance productivity, integrating with our existing applications like email and non-public data such as company intranets, to make finding answers and creating output much easier.
We are at the very beginning of the GenAI journey, and much is apt to change in the months and years ahead. As a Fortune 100 CTO recently described it to us, “if GenAI is a mile long race over the next 5 to 10 years, at this point, we have travelled only 10 feet.”
GenAI Technology Stack
We think the AI stack (or framework) developed by Amazon CEO Andy Jassy, as seen below, lays out the GenAI technical ecosystem well.
Layers | What’s important | Examples (not exhaustive) |
---|---|---|
Applications | Ability to reach users in an accessible way | ChatGPT, Google Search Generative Experience, Snapchat MyAI, Meta AI |
Models and data | Large language models (LLMs), foundational models (FMs), large proprietary datasets | Models: GPT-4 (OpenAI), Llama (Meta), Gemini (Alphabet) Datasets: e-commerce transaction data (Amazon), search queries (Alphabet) |
Compute and infrastructure | Scale and competitive chips | Infrastructure: AWS (Amazon), Azure (Microsoft), GCP (Alphabet) Semiconductors: NVIDIA, Alphabet, Amazon |
At the bottom of the stack, and the foundation of all AI, we have compute and infrastructure, specifically cloud computing (as primarily offered by Amazon’s Amazon Web Services (AWS), Microsoft’s Azure, and Alphabet’s Google Cloud Platform (GCP)) and AI-specialized chips (most commonly offered as GPUs, largely by NVIDIA). In addition to its chips, NVIDIA has entered the compute arena with NVIDIA DGX™ Cloud, which gives businesses access to the infrastructure needed to train advanced models for GenAI.
In the middle of the stack, we have the GenAI models, including large language models (LLMs), which are more language specific, and foundational models (FMs), which are more general purpose, and the data these models consume. Many companies are developing their own models, including OpenAI with GPT-4, which powers ChatGPT; Alphabet with PaLM, which powers Google Bard and soon-to-be-released Gemini; Amazon with Triton; NVIDIA with its library of pre- trained models; and Meta with Llama. We also expect to see any number of startups whose models will be available on the three hyperscalers — the dominant large cloud service providers Amazon, Alphabet, and Microsoft.
At this time, we believe companies will use different models for different use cases, with most using a mix of LLMs and FMs depending on the application. However, because the efficacy and strength of a model is heavily dependent on the data it is trained on, companies with unique, commercial proprietary data at scale will have an advantage, as we explain in more detail below.
At the top of the stack is the application layer, where consumers and enterprise employees interact with GenAI applications. The most famous GenAI-powered application to date is ChatGPT, which reached 100 million users in only two months after it became publicly available in November 2022. We believe the use of large-scale applications like ChatGPT and Google’s version, Bard, will increase significantly, as Microsoft (which is partnered with Open AI, ChatGPT’s owner) and Alphabet leverage their vast distribution advantage to reach a growing consumer and enterprise customer base. In addition to ChatGPT-type general chat-focused applications, we will see chatbots embedded into most existing commercial websites, mobile applications, and e- commerce sites. In this paper, we focus on the consumer application layer, where we believe we will see the most dramatic change in the shortest timeline.
Consumer Application Layer: Introduction to AI Agents
We believe GenAI has the potential to serve as a dedicated agent or personal aide, transforming our online interactions and the way we retrieve information, manage everyday tasks, and even create content.
Currently, we spend a significant amount of time navigating through websites and apps to find what we need. For tasks like planning a trip or buying a product or service, the process typically involves multiple steps: searching on platforms like Google, browsing several sites, reading reviews, comparing prices, and, finally, making a purchase (or not). This method is time-consuming and often inefficient.
When correctly trained and integrated with existing applications, a GenAI tool can essentially function as a personal assistant. With consistent usage, this AI agent, adapting to an individual’s preferences, will be able to efficiently handle a plethora of online tasks, from ordering groceries to drafting emails to planning a vacation. Over time, the AI agent’s predictive capacity should evolve, much like a seasoned human assistant who anticipates needs. For instance, recalling a user’s penchant for unique Mother’s Day gifts, the AI agent might proactively offer gift options personalized for the user.
This technology also holds the promise of boosting work productivity and offers capabilities previously inaccessible to many individuals. Workers could (and have already started to) use GenAI to develop code, create marketing campaigns, or generate a lesson plan. For instance, small business owners could tell GenAI to code and design a website for their business using pictures of them and their LinkedIn page in a modern style with a blue color palette. (You can try a version of this yourself at durable.co, or a number of other startups, that uses AI to build custom websites in roughly 30 seconds.)
“In five years, everybody [will] have their own [AI] ‘chief of staff’ ... that will intimately know your personal information, be completely aligned with your interests, and help you manage and process all the information you need.”
— Mustafa Suleyman, co-founder and ex-head of applied AI at Google DeepMind.
We posit that proficient AI agents are likely to eventually surpass traditional methods of online browsing and searching, primarily due to their enhanced precision and time-saving attributes. Earlier iterations of virtual assistant technology, such as Siri, Alexa, and Google Assistant, although innovative, do not yet reach this envisioned level of accuracy and personalization. While immediate widespread adoption of AI assistants might be a stretch, we are confident that, within the next decade, a robust and universally embraced version of AI agents will emerge.
Consumers | |
---|---|
Today | In Five Years |
• Employ search engines and click on multiple links • Go directly to multiple apps and websites for different use cases | • Employ a few general and best-of-breed agents that will integrate with shopping, email, entertainment, etc. to perform a multitude of tasks/functions • Visit fewer websites and apps • Spend less time searching and browsing for answers and products |
The widespread proliferation of AI agents and GenAI-informed consumer applications has many ramifications and raises a number of interesting questions. High-level examples of how this technology could change industries and actions include the following:
Travel
Rather than searching through travel sites, YouTube videos, and Instagram to plan trips, a person could use a consumer-friendly application that knows that person’s flight, hotel, and activity preferences. The person could type or say, “plan a Labor Day weekend trip to New Orleans,” and be able to book and plan everything in 15 minutes through a single chat-based interface that could prompt questions about the trip and personal preferences as it goes through the process.
On the back end, the app would partner with (or be a part of) travel-related providers such as airlines, hotels, restaurants, and tours to help optimize the trip itinerary based on data, pricing, and availability.
Entertainment
A person or business could request or create GenAI-generated/aided content that takes entertainment preferences into account. We already have seen some early examples, such as an AI-generated song using the voices of Drake and the Weeknd that went viral earlier this year. While entertainment companies will need to address copyright and distribution-related concerns, we believe they will be incentivized to lean into consumer preferences and build on their existing intellectual property (IP). For example, Tencent, the world’s largest video game company, has stated it intends to explore the use of GenAI to develop immersive virtual worlds with more interactive non-playing characters and more dynamic environments using existing IP.
Commerce
GenAI could serve as a shopping assistant, consolidating the search, browse, and purchasing steps into one. Imagine Alexa not only helping you find a winter jacket but also reading to you a summary of online reviews (in fact, Amazon is already using GenAI to summarize reviews).
Competitive advantages of GenAI consumer applications
The two primary sources of competitive advantage for consumer applications in a GenAI world are large proprietary datasets and scaled distribution.
Large, proprietary datasets
Large, proprietary data sets allow AI agents or models to gain unique insights specific to the training of these agents or models. For example, Amazon, as the top e-commerce website with more than half of the industry’s net sales, has the world’s largest dataset of e-commerce transactions.
Alphabet’s dataset is built on its dominance in search— Google handles 90% of all search queries worldwide, YouTubers spend an average of 45 minutes per day on the service, and Google Maps is the dominant mapping service. All these services provide proprietary data around intent, interests, behavior, and location involving daily user activity.
Meta’s dataset of billions of daily views garners information on user friends, family, interests, events, commercial interests, and groups and relevancy of ads. We believe GenAI can leverage these large datasets to provide hyper-personalized recommendations. For example, we estimate Meta serves over 50 trillion ads to consumers each year. As a result, Meta has substantial data on what ads look like, who they are shown to, and how users interact with them. With this vast and proprietary dataset, Meta could offer different iterations of ads customized for a given consumer. While online ads have always been targeted, they can become even more targeted, with the creative copy and imagery optimized to what the data suggests a particular individual will best respond to.
Scaled distribution
Scaled distribution allows companies to reach a large customer base cost effectively and use the reinforcement learning from that base to improve the core AI agent or model.
While startups and other entrants will certainly seek to compete in the space, large incumbents should have an advantage by pushing GenAI agents to their massive customer bases. Alphabet has nine apps with over one billion users each; Meta has three apps with over two billion users each; and Amazon is, in many ways, synonymous with online shopping. The benefits of scale, billions of users interacting with these models, and the ability to capture the learnings from these interactions, will make these large-scale models even more informed and ultimately more accurate. We believe Alphabet already has over 100 million users of Google Bard (Alphabet’s version of ChatGPT) and is using those interactions to inform and improve their foundation models.
For these reasons, we think the widespread use of GenAI agents will likely result in further consolidation of consumer applications to the largest platforms in each category given their advanced data and distribution capabilities.
While GenAI will benefit the companies able to maximize its potential, the disruptive impact of this technology will present a challenge to those that do not or cannot. For example, the mid- and long-tail of the website universe could face pressure if the GenAI agent offers just one or two potential options from the dominant websites instead of the multitude that a search produces today.
Four Companies to Watch
We are in the early innings in the GenAI revolution. While a handful of companies have demonstrated GenAI capabilities, these features are still viewed as novel and are essentially unmonetized. However, we believe this will change dramatically over the next three to five years and beyond.
We see four companies in particular – Alphabet, Meta, Amazon, and Tencent1 — that are optimally positioned to profit from GenAI in the consumer application space. While all four are well known mega-cap technology companies, we believe the tangible GenAI impact of these companies has yet to be fully appreciated.
To be sure, several of these stocks have run up year-to-date, but we believe this increase was largely attributable to catch-up after a brutal 2022 coupled with demonstrated earnings power rather than any material financial contribution from GenAI.
Each has large, unique, proprietary datasets, massive distribution, and strong technical infrastructure and talent. They also have significant cash reserves and sources of funding for potentially expensive AI ventures.
COMPETITIVE ADVANTAGES | |||
---|---|---|---|
Data | Distribution | Technical Access | |
Alphabet | • Search:significant transaction visibility; 90% of broad based search engine activity; browsing data and patterns • YouTube: 800M+ videos • Google Maps and Waze and Google Flights and Google Hotels travel data • Display ad network data • Large internal codebase | • 9 products with >1 billion users: Search, YouTube, Android, Chrome, Gmail, Google Drive, Google Maps, Play Store, Google Photos. They also have Nest and other home and assistant devices • Universally known and widely trusted brand • Sizable existing advertising base | • Google Cloud infrastructure • Hyperscaler with NVIDIA GPU access • Developing stronger inhouse TPU chip capability • Strong AIresearch center (Google DeepBrain) • Top engineering talent base |
Meta | • Largest globa lsocial network with data on individuals, preferences, likes, etc. • Some visibility into transaction data through advertiser integrations • One of the most popular short video platforms with > 200 billion daily views | • >2 billion users each on Facebook, Instagram, WhatsApp, >1 billion on Messenger • Universally known brand • Sizable existing advertising base | • Hyperscaler with NVIDIA GPU access • Strong AI research center with demonstrated open-source models and applications • Top engineering talent base |
Amazon | • Largest ecommerce company with huge transaction dataset • Large and growing video business • Large and growing advertising business | • Massive third party retailer base • Universally known brand • Home and assistant devices (Alexa) | • AWS cloud infrastructure • Hyperscaler with NVIDIA GPU access • Developing inhouse chip capability (Tranium, Inferentia) • Top engineering talent base |
Tencent | • Largest video game company in China • Largest social media company in China • One of the two largest payments companies in China • Large short video platform | • >1 billion users across Weixin and WeChat • Universally known in China • Strong government ties (more likely to get approvals) | • Tencent Cloud infrastructure • Government-approved to use GenAI in apps • Very strong engineering talent base |
GROWTH OPPORTUNITIES | |||
---|---|---|---|
Alphabet | Meta | Amazon | Tencent |
Revenue | |||
• More meaningful entry into e-commerce • More involved travel assistance • Productivity assistance in Gmail and Google Workspace • AI content creation for YouTube/entertainment • AI-optimized dynamic ads | • More meaningful entry into effective search through chat apps (including WhatsApp overseas) • Opportunity to enter e-commerce through consumer-facing AI agent • Monetize layers and licenses on top of open source • AI-optimized dynamic ads | • Even greater ecommerce penetration with AI agent, less to long tail of stores • Could enter search through general assistant applications • Content creation in Prime Video • AI-optimized dynamic ads | • Enter search as a chatbot through its massive chat applications • Could enter e-commerce more broadly through chatbot • Increase in video game production, particularly for existing IP • AI-optimized dynamic ads |
Costs | |||
• Reduced code creation costs • Reduced content creation costs • Higher standard corporate efficiencies |
Alphabet
Alphabet has massive distribution, perhaps the largest set of consumer data (through search, transactions, and other use cases), and strong technical infrastructure. It has a strong history of investing in and leading AI research, famously through its labs DeepMind and Google Brain.
We are aware that Alphabet’s dominant search business has the potential to experience some disruption as other companies roll out AI agents that seek to offer chat and search-like functionality.
However, we believe Alphabet will maintain its leadership role due to its dataset advantage derived from having over 90% of broad search queries, interactions with an estimated four billion consumers globally, and scaled and highly specific infrastructure to provide search-like results. Alphabet’s dataset advantage also reaches well beyond search into domains such as maps, images, videos, audio, home devices, mobile phones, travel, and retail.
Google Search Generative Experience, a GenAI agent in beta, is already demonstrating how Alphabet can substantially improve search results through GenAI. At the same time, competitors are still trying to catch up to Google's traditional search capabilities.
Google Bard, a GenAI chatbot, has been providing real-time results for months, while we await the next iteration of GPT-4, which is trained on data that does not extend past September 2021 (though it has a Bing integration to provide real-time answers). Google also is working on its Gemini model, which is expected to be multi-modal (e.g., supports images as well as text) and better at logic and creativity.
On the enterprise side, Alphabet introduced Duet AI for Google Workspace, a GenAI tool that helps workers write, organize, visualize, accelerate workflows, have more productive meetings and other work-related functions. We also think Google Cloud (both infrastructure and applications) will become more useful with GenAI.
Meta
Meta has the largest global social network with 3.8 billion monthly active users across its apps, the unique data and relationships that this network provides, and a strong base of engineering talent.
We believe Meta can leverage its leading position in social networks Facebook and Instagram and messaging apps WhatsApp (two billion users) and Messenger (one billion users) to offer more enhanced AI agents, encroaching on search.
The text box in any of these apps is a logical entry point to provide a prompt base for a search-like experience when a user starts to enter a text or message. The explore tab in Instagram is another. In late September, Meta announced its plans to offer multiple AI assistants for different use cases, like personal training, cooking, or general advice. Eventually, we expect Meta to leverage this real estate to become a more meaningful e-commerce player through the use of AI agents, although it is still a question whether Meta has enough data on merchants, inventory availability, and the SKU taxonomy to execute on this pote ntial.
On a technical level, Meta is one of the largest purchasers of NVIDIA’s highest quality chips, has the leading open-source AI lab, and has a strong base of top engineering talent. Meta’s Llama models are considered the best open-source GenAI models available on the market today. We believe its use of recommender systems from NVIDIA has already helped Meta provide more targeted advertising through customized ads and better engagement through personalized content. Meta’s work on short videos, along with AR and VR, is ongoing.
Amazon
Amazon is the world’s largest global e-commerce player with substantial market penetration, including 40% of U.S. e-commerce, generating scaled, highly valuable transaction data. It also has leading cloud and technical infrastructure through AWS.
Currently, consumers see the impact of AI through product recommendations (“you may also like”) and review summaries. We think Amazon can grow its e-commerce share even more through a consumer AI agent, which could also be used for broader search queries. Behind a more intuitive front-end agent (which others can also offer to some extent), Amazon’s strong item inventory data and leading fulfillment and shipping capabilities should make the overall GenAI shopping experience easy and reliable.
We also see potential opportunities tied to Prime Video through an entertainment AI agent. Its virtual assistant device Alexa presents another opportunity to reach consumers through an AI agent.
With the best attribution of any advertising provider due to its ability to keep consumer searches and purchases within its platform, Amazon could leverage GenAI to offer more targeted advertising.
We believe Amazon is also reasonably positioned in the enterprise space through AWS, which companies could use to build their AI stacks on top of their existing cloud infrastructure. It is still early, but with the right partnerships (such as with leading AI model startup Anthropic or with Meta for its Llama model), Amazon can provide ready access for enterprises to use AI.
Tencent
Tencent is the leading video game and social media company in China with over one billion users and formidable data and distribution capabilities. The company also has a substantial cloud infrastructure division.
Tencent currently uses recommendation-based AI, including in its increasingly popular short-form video content feature, and is testing its GenAI model Hunyuan with its enterprise clients.
We believe Tencent will use its channels, particularly its messaging, social media, and electronic payment app WeChat, to introduce a consumer AI agent that will try to compete in the search market, long held by Baidu. We also acknowledge the risk of constraints on chip exports to China potentially impacting Tencent’s GenAI buildout.
We see opportunities in other existing and new markets as well. For instance, Tencent could use GenAI to make video game content much more personalized and extensive. It may also leverage GenAI to enter the e-commerce/travel segments, possibly with a partner if specialization and significant complexity is involved. Like its U.S. counterparts, Tencent AI can also offer more personalized advertising through GenAI.
Conclusion
In the epoch of the digital revolution, where data is invaluable and distribution is pivotal, companies like Alphabet, Meta, Amazon, and Tencent have emerged as influential leaders in the technological landscape. These corporations wield notable competitive advantages, enabling them to flourish in the present and strategically position themselves for a prosperous future.
Alphabet’s expertise in organizing information, Meta’s proficiency in fostering social connections, Amazon’s acumen in e-commerce, and Tencent’s versatility in online services have enabled them to intertwine deeply in the global digital tapestry. Armed with vast reservoirs of proprietary data, they navigate the realms of innovation and adaptation with unparalleled dexterity, ensuring their offerings resonate with evolving consumer needs and preferences. Through the harnessing of comprehensive distribution networks and the astute application of their data insights, these companies stand poised, not just as participants, but as formidable architects of the forthcoming waves of technological and market advancements.
Glossary
Large language model (LLM) is a type of artificial intelligence (AI) algorithm that uses deep learning techniques and massively large data sets to understand, summarize, generate, and predict new content. LLMs are trained on datasets of text and code typically containing billions or even trillions of parameters. This allows them to learn the complex patterns and relationships that exist in language. They can be used for a variety of tasks, including text generation, translation, summarization, responses to questions, natural language inference, and sentiment analysis. LLMs are still under development, but they have the potential to revolutionize the way we interact with computers. They could be used to create more natural and engaging userinterfaces, to generate more creative and informative content, and to improve our understanding of the world around us.
Foundational model (FM) is a large machine learning (ML) model trained on a vast quantity of data at scale, often by self-supervised learning or semi-supervised learning, that allowsit to be adapted to a wide range of downstream tasks. FMs have helped bring about a major transformation in how AI systems are built by powering prominent chatbots and other user-facing AI. They are trained on massive amounts of unlabeled data that allows them to learn general patterns and relationships rather than being specifically trained for a particular task. They are very large and complex which allows them to capture the nuances of the real world and to perform tasks that would be difficult for smaller models. They are also adaptable and can be fine-tuned to perform a specific task, even ifthey were not originally trained forthat task. Examples of foundation models include GPT-3 and GPT-4, which were developed by OpenAI, BERT, which was developed by Google AI, and DALL-E 2, an image modeling framework developed by OpenAI as well. FMs are still under development, but they have the potential to revolutionize the way we interact with computers. They could be used to create more natural and intuitive user interfaces, develop new medical diagnostics, and improve our understanding of the world around us. The primary difference between deep learning and FMs is the amount of data they are trained on. Deep learning models are typically trained on smaller datasets, while foundation models are trained on massive datasets. This allows foundation models to learn more general patterns and relationships, which makes them more adaptable to a wider range of tasks.
Graphic processing unit (GPU) is the primary chip used for AI workloads. Originally designed for rendering graphics, GPUs have evolved to excel at parallel processing with their thousands of small cores. This makes them ideal for tasks that can be split and processed simultaneously, including ML as it is used in AI. NVIDIA is currently the leading manufacturer of GPUs, with 90% of the market, although AMD and the hyperscalers have competing chips. GPUs differ from central processing units (CPUs), which are used for general purpose, more versatile tasks. CPUs typically possess fewer cores than GPUs and are more adept at sequential, rather than parallel, processing.
Hyperscaler refers to the very large cloud service providers, namely Amazon Web Services (AWS), Microsoft Azure (Azure), and Google Cloud Platform (GCP). These companies provide infrastructure-level cloud computing and storage as well as various platform and application cloud services.
Reinforcement learning human feedback (RLHF) is a ML method in which the reinforcement learning model is trained using feedback from humans. This feedback can be in the form of demonstrations, comparisons of different actions, or direct corrections to the model’s behavior. The purpose is to improve the performance and safety of reinforcement learning algorithms by incorporating human judgment and expertise. It is commonly used in scenarios where traditional reinforcement learning might be unsafe or inefficient, or when the desired behavior is difficult to specify using a reward function alone.
Featured Fund
Learn more about Baron Technology Fund.
Baron Technology Fund
- InstitutionalBTECX
- NAV$11.98As of 04/25/2025
- Daily change1.87%As of 04/25/2025